Hello, dear reader, and welcome to another issue of AI, Law, and Otter Things! Today I want to share with you a brief excerpt that I ended up cutting from my thesis. In the following paragraphs, I offer some thoughts on the ages-old question: how can one conduct legal research that engages with technology? After all, both the law and the technical aspects of technology are complex enough on their own. My point here is by no means a stab at a final word about this topic, but it might be interesting to some of you, if only for the references.
In one way or another, the issue of “how to deal with tech?” has been a central question from the early days of my PhD. But, at the end of the day, my thesis ended up evolving in a different direction than the one that originally motivated me to write these paragraphs. Back then, I was trying to propose tools to allow legal scholars to look more closely into the technical side of things without necessarily becoming legal technologists.1 Nowadays, however, I am more interested in understanding the kind of institutional arrangements that are needed to make technology-neutral regulation possible in the first place. Accordingly, I dropped my initial efforts towards developing methods for interdisciplinary collaboration. I still believe that is a necessary task, so I share these notes in the hopes they might be useful to someone.
Any argument that regulation must deal with the technical aspects of AI must address a simple question that is by no means easy: how to do so? Considered as a class, legal scholars and policymakers are not particularly acquainted with the technical aspects of AI, and scholars from the technical disciplines are not well-versed in the issues and methods of regulatory studies.2 As a reflection of this gap, the last few years have seen a boom in interdisciplinary collaboration, which translates into academic publications with authors from various disciplines3 and venues to publish work on the interfaces between technology and regulation.4
The portraits of AI technologies produced by these practices might be distorted to a lesser or greater degree by some practical considerations, such as the differences in technical practices between software development in academia and the industry5 or the growing concentration of AI research in corporate labs to the detriment of universities.6 Nonetheless, the expertise available within computer science departments and related disciplines offers various possibilities for bridging the technical knowledge gaps in regulation scholarship.
As an alternative—or sometimes as a complement—to collaboration with technology scholars, legal and regulatory scholarship has also drawn on the methods of the social sciences. For example, Gavin Sullivan proposes that AI technologies and other decision-making systems should be approached in terms of their infra-legalities, that is, of the infrastructural configurations that shape and render possible certain uses of technology for the law.7 Approaches like these draw from methods and concepts used in science and technology studies, such as infrastructures,8 actor-network theory,9 and affordances.10
By combining these tools with established legal ones, scholars can avoid subordination to the “black box” metaphor. Looking at AI systems not just in terms of their technical properties but as broader socio-technical systems allows legal scholars and policymakers to examine features of these systems beyond their technical innards. They can, for example, engage with how the system interfaces with the world.11 A socio-technical view also allows scholars and policymakers to identify the constraints and aims that shape technical decision-making. Socio-technical approaches to AI technologies and their regulation can provide valuable insights into how these technologies are used in the real world.
Yet, the combination of socio-technical approaches to AI and interdisciplinary work does not address all the epistemic needs of regulation. Situated analyses of AI systems provide data about what AI can and cannot do in the real world and about how it interacts with that world. But laws are meant to have a general effect,12 and so policymakers must find common ground between the various situations regulation is meant to govern.13 In the case of technology-specific regulation, policymakers must identify which aspects of technology are relevant to their regulatory aims, making judgment calls that scholars can assess in their broader evaluation of the regulatory approach. To do so, they often rely on metaphors: debates about internet regulation, for instance, are strongly shaped by the cyberspace metaphor.14
A few metaphors have been particularly salient in AI-related discourse, notably the use of “black box” as a shorthand for the various forms of technical and social opacity surrounding AI systems. Conceptual research in AI regulation can identify new metaphors that help policymakers and scholars make sense of sociotechnical complexities.15 It can also provide critiques of established metaphors and suggest potential alternatives that are fitter for the purposes they play in regulation.16 Hence, conceptual work can supply AI regulation debates with sense-making tools that can be used to guide further empirical work.
Other conceptual tools can supplement or even extend the reach of technological metaphors. One such tool is the use of representational models, which are used as a stand-in that emphasizes certain relevant properties of the object under analysis. This is an idea that I explored in a recent issue of this newsletter, so you might want to check it out. But how can one find which models can be used to address a particular technological issue? As Ronald Giere pointed out, models are interest-specific, so the answer will depend on the purposes of the analysis. Sometimes, it can be useful to use an established model as a starting point for making sense of regulation. But one might also try to find whether the law already reflects a particular model, following what one might call a reverse engineering approach.
Some legal scholars have already proposed the use of reverse engineering methods. For example, Michael Birnhack shows how one can identify the “technological mindset” that unavoidably is reflected in technology-neutral regulation.17 Elsewhere, Fabrizio Esposito proposes a reverse-engineering method to identify the concepts that are materialized in regulatory arrangements.18 Such an approach has an affinity with the use of representational models, as a model can be used to articulate what the law presupposes to be true about technical objects or processes.
The reconstructed model can, then, be used for various purposes. It can guide the interpretation of existing legal provisions,19 be used to highlight differences between the concepts present in the legal text and those used in practice, or provide a starting point for critiques of the established concept. These and other uses suggest that, even though debates about the effectiveness of technology-specific approaches are ultimately empirical, they can be enriched by an adequate treatment of the underlying concepts.
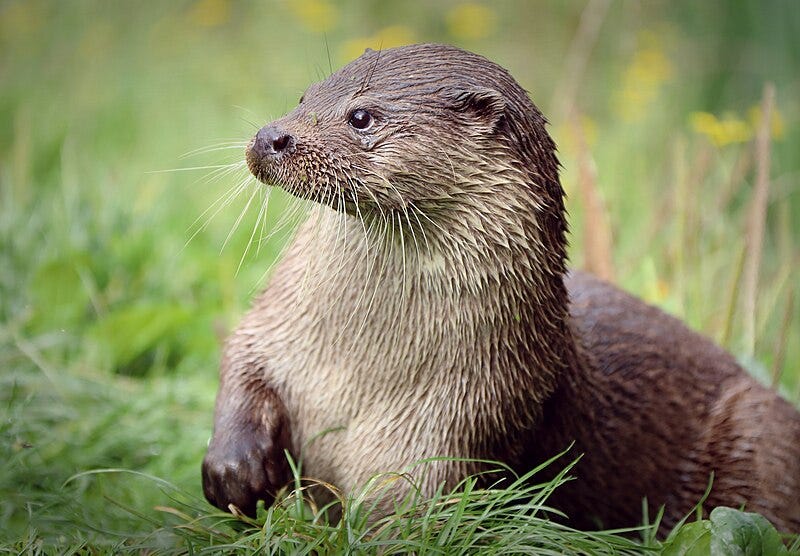
Thanks for reading! Please consider subscribing if you haven’t done so yet, and I hope to see you for the next issue!
Spoiler alert: this involved finding good methods for collaboration with experts from various domains.
Occasionally, some scholars have been educated on both sides of the divide. This is not only my case, but also that of more established scholars such as Paul Ohm, and a dual background is increasingly common in the United States due to their approach to legal education as a graduate course of studies. A dual formation can be a powerful asset, but it also introduces certain constraints on how one approaches legal studies. I will not elaborate further on these points for a simple reason: it is not sustainable to expect that every legal scholar needs to be a computer scientist (or any other formation), as that would come at the expense of depth in either discipline. See, e.g., Mireille Hildebrandt, Law for Computer Scientists and Other Folk (Oxford University Press 2020) 1.
See, for example, the vast interdisciplinary literature on algorithmic transparency that I discuss here.
See, e.g., journals such as the Journal of Cross-disciplinary Research in Computational Law (CRCL) or conferences such as the ACM Conference on Fairness, Accountability, and Transparency (ACM FAccT).
See, e.g., Dusica Marijan and Sagar Sen, ‘Industry–Academia Research Collaboration and Knowledge Co-Creation: Patterns and Anti-Patterns’ (2022) 31 ACM Transactions on Software Engineering and Methodology 1.
Nestor Maslej and others, ‘The AI Index 2023 Annual Report’ (HAI 2023).
Gavin Sullivan, ‘Law, Technology, and Data-Driven Security: Infra-Legalities as Method Assemblage’ (2022) 49 Journal of Law and Society S31.
Leticia Barrera and Sergio Latorre, ‘Actor-Network Theory and Socio-Legal Analysis’ in Mariana Valverde and others (eds), Routledge Handbook of Law and Society (Routledge 2021).
Benedict Kingsbury, ‘Infrastructure and InfraReg: On Rousing the International Law “Wizards of Is”’ (2019) 8 Cambridge International Law Journal 171.
Laurence Diver, ‘Law as a User: Design, Affordance, and the Technological Mediation of Norms’ (2018) 15 SCRIPTed 4.
Till Straube, ‘The Black Box and Its Dis/Contents: Complications in Algorithmic Devices Research’ in Marieke de Goede, Esmé Bosma and Polly Pallister-Wilkins (eds), Secrecy and Methods in Security Research: A Guide to Qualitative Fieldwork (Routledge 2019).
On generality as a central property of the law, see, inter alia, Gregor Kirchhof, ‘The Generality of the Law: The Law as a Necessary Guarantor of Freedom, Equality and Democracy and the Differentiated Role of the Federal Constitutional Court as a Watchdog’ in Klaus Meßerschmidt and A Daniel Oliver-Lalana (eds), Rational Lawmaking under Review: Legisprudence According to the German Federal Constitutional Court (Springer 2016); Fernanda Pirie, ‘Beyond Pluralism: A Descriptive Approach to Non-State Law’ (2023) 14 Jurisprudence 1.
Sometimes, regulation can be very specific: for example, some of the provisions in the EU interoperability regulations are used to specify properties and requirements for the systems that must be implemented as components to facilitate interoperability between the various databases and systems operated by Member States in the domain of the Area of Freedom, Security, and Justice: see, e.g., Alexandre Au-Yong Oliveira, ‘Recent Developments of Interoperability in the EU Area of Freedom, Security and Justice: Regulations (EU) 2019/817 and 2019/818’ (2019) 5 UNIO – EU Law Journal 128. Most technology-specific rules, however, are directed at entire classes of systems, or even more broadly-construed technologies.
Julie E Cohen, ‘Cyberspace as/and Space’ (2007) 107 Columbia Law Review 210; Ira Steven Nathenson, ‘Cyberlaw Will Die and We Will Kill It’ in Sharon Sandeen, Christoph Rademacher and Ansgar Ohly (eds), Research Handbook on Information Law and Governance (Edward Elgar 2021).
See, e.g., Bhargavi Ganesh, Stuart Anderson and Shannon Vallor, ‘If It Ain’t Broke Don’t Fix It: Steamboat Accidents and Their Lessons for AI Governance’ (2022).
See, e.g., Straube, ‘The Black Box and Its Dis/Contents’.
Michael D Birnhack, ‘Reverse Engineering Informational Privacy Law’ (2012) 15 Yale J L & Tech 24.
Fabrizio Esposito, The Consumer Welfare Hypothesis in Law and Economics: Towards a Synthesis for the 21st Century (Edward Elgar Publishing 2022) ch 4.
This is, for example, how Esposito uses the method: to argue that EU antitrust and consumer law should be understood as seeking to maximize consumer welfare rather than total welfare.